Introduction
Firstly, a brief background about myself. I am working as a Software Engineer in one of the Alternate Asset Management Organization (Handling 1.4 Trillion with our product suite) responsible for maintaining and developing a product ALT Data Analyzer. My work is focused on making the engine run and feed the machines with their food. This article explains the problems we faced with scaling up our architecture and solution we followed. I am dividing the blog in the following different sections:- Product Brief
- Current Architecture
- Problem With Data loads and Sleepless Nights
- Solutions Tried
- The Final Destination
Product Brief
The idea of building this product was to give users an aggregated view of the WEB around a company. By WEB I mean the data that is flowing freely over all the decentralized nodes of the internet. We try to capture all the financial, technical and fundamental data for the companies, pass that data through our massaging and analyzing pipes and provide an aggregated view on the top of this data. Our dashboards can be one stop for all the information around a company and can aid an investor in his analysis.
Some of the Data Sources that we support as of now are:
- Stock News from multiple sources
- RSS feed
- Yahoo News
- Yahoo Finance
- Earning Calenders
- Report Filings
- Company Financials
- Stock Prices
Current Architecture

Broad View
We knew that problem that we are solving has to deal with the cruel decentralized Internet. And we need to divide the large task of getting the data from the web and analyzing it into small tasks.

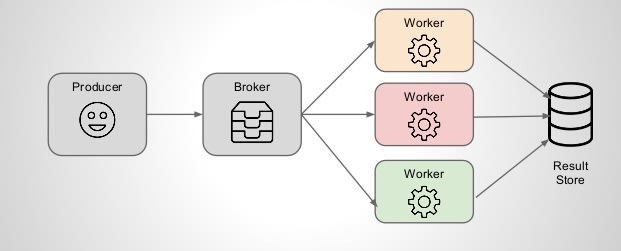
Problem With Data loads and Sleepless Nights
Everything was working fine with the development workloads, we had deployed around a cluster of 5 machines in the celery cluster (i7 processors with 16 gigs of memory each) with each machine running some celery workers.
But this was the calm before the storm. As we planned to increase our workloads and configure more tasks the system started to fade and run out of memory very frequently. We were now generating around 10 million tasks a day. Celery workers were not able to process the tasks at this speed and this was building up tasks in the queues which were slowing down the Redis broker as it was now storing a large number of tasks, this was, in turn, making the whole system run out of memory and gradually to a halt.
Our beautifully designed ship was sinking and we had to do something to save it.
Solutions Tried
As we faced this issue the first approach we thought, as any other developer would have applied is to add more nodes to the cluster. While discussing this solution there was an argument that if the loads increases again with time, will we be adding more nodes again and again.
So, we skipped this solution and agreed to dig deep into the problem.
On deep analysis we realized that a large number of tasks were populated by the publishers and these tasks were getting stored in the Redis queues, with the data loads the Redis eventually slows down the rate at which the tasks were being sent to the consumers making the consumers idle.
So our system was stuck in an infinite loop.
- Redis sends tasks at high speed but consumers cannot consume at that rate.
- Redis slows down and lowers the rate at which it sends tasks and now consumers stay idle most of the time.
In both the situations, the common name that was in doubt was the Redis DB. On researching and exploring the RedisDB we found that it is a single-threaded system and performs all the tasks in a round robin fashion. Also, it was saving the tasks received by the systems in memory which was increasing the memory consumption and the under heavy loads that single thread was busy performing the persistence and other tasks that it slows down the polling process initiated by the consumers. So, we found the reasons for both the problems that we were facing.
To handle the heavy workload one of the choices was to shard the Redis server into a cluster. So we created a Redis partition set (tutorials can be seen here). We created a cluster of three nodes with each node handling an equal number of keys through a consistent hashing technique.
We plugged this cluster with the celery app and the workers were throwing an exception "MOVED to server 192.168.12.12"
On googling we found that Redis cluster is not yet supported by celery. On one hand we thought we had a solution but on the other, that was not yet supported by the underlying framework :(
Now the exploration began again to get a solution to our problem and we thought of using a proxy server in front of Redis cluster Twemproxy. But this time we first choose to check the compatibility with our framework and boom...... we cannot be more wiser in taking this path.
Proxy was not yet supported by Celery.
Now frustrated with this incompatibility issue we tried to figure out what all things are compatible with the framework. Some of the compatible brokers were
- SQS
- Redis
- RabbitMQ
- Zookeeper
A straightaway thought was to try a different broker so, we began to explore these options. Following table proved useful in narrowing down our research
Name | Status | Monitoring | Remote Control |
RabbitMQ | Stable | Yes | Yes |
Redis | Stable | Yes | Yes |
Amazon SQS | Stable | No | No |
Zookeeper | Experimental | No | No |
Redis is what we have already tried so we went for the second option that is stable and provides more features i.e RabbitMQ.
Spoiler:
By now we knew that RabbitMQ is one the best choice for the brokers and is used by wide variety of clients in production and Redis is the best choice in terms of result backend (intermediate results that are stored by a task in Celery chains and chords).
We did necessary changes to the system and tried to run the system.
This time system nearly went into an irrecoverable state consuming all the memory and we had to restart the servers.
While all this was happening we were monitoring the RabbitMQ through its admin tool and found a fishy thing. It was creating a large number of queues nearly as many as the number of tasks.
On googling we found that the queues were created to store the result of the intermediate tasks and this was consuming too much space in the memory as all this storage was in memory.
The Final Destination
Collecting all the clues from our exploration we decided to use both the systems i.e Redis and RabbitMQ for the work that they are best at.
We deployed Redis as a result backend to store the intermediate results and RabbitMQ as a broker for maintaining communication and passing tasks (remember the spoiler above).
With this architecture, we were able to run the system under the workload on over 10 million tasks a day which can be scaled easily.
Hope this helps someone going through the same problem.
Acknowledgments
We took hints from the Celery documentation, Redis documentation, Stackoverflow threads, Github Issue page of Celery, Case studies by Instagram and Trivago.
Top comments (1)
Hi you have written this post in 2018,
Now this is 2021, did celery made any changes for the MOVED '172.33.0.0' something like that exception?
Because I am also getting error.
Or can you show me the example for the above post?
Waiting for the response soon....
Thanks in advance.